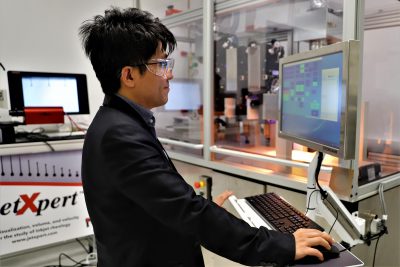
Inkjet printing has evolved from a graphics and marking technology to a broader variety of additive manufacturing and 3D printing processes for electronic, optical, pharmaceutical, and biological applications. The success of adopting inkjet technology for these newer applications is contingent on whether the ink materials can be consistently and reliably jetted by the print systems. Currently, each printer-and-ink combination requires calibration by trial and error, which consumes a considerable amount of time and materials. IPB researcher, Prof. Anson Ma, Site Director of SHAP3D, teamed up with UConn machine learning expert, Prof. Qian Yang, to demonstrate a new concept of “autonomous 3D printing”, leveraging an active machine learning method they developed to efficiently create a jettability diagram that predicts the best conditions for jetting an ink from a printhead.
Briefly, a camera is used to image the printhead and capture the behavior of ink jetted from a printhead. Starting with a few randomly chosen conditions, a machine learning algorithm predicts the optimal jetting conditions and then “cleverly decides” on the next set of experiments that can further improve prediction accuracy. After performing those experiments, the algorithm analyzes the newly acquired images, updates the prediction for the desired jetting conditions, and iteratively selects the next experiments, continuing autonomously until a small experimental budget is reached. This approach has achieved a prediction accuracy of more than 95% while considerably reducing the number of experiments required by 80% compared to a typical grid-search approach. This novel approach is especially powerful for optimizing complex print systems with many tunable process parameters.
This work was recently published in the journal 3D Printing and Additive Manufacturing (http://doi.org/10.1089/3dp.2023.0023) and led to a pending patent application (WO 2023/2788542).